Feed Your AI Algorithm with Clinical Quality Measures
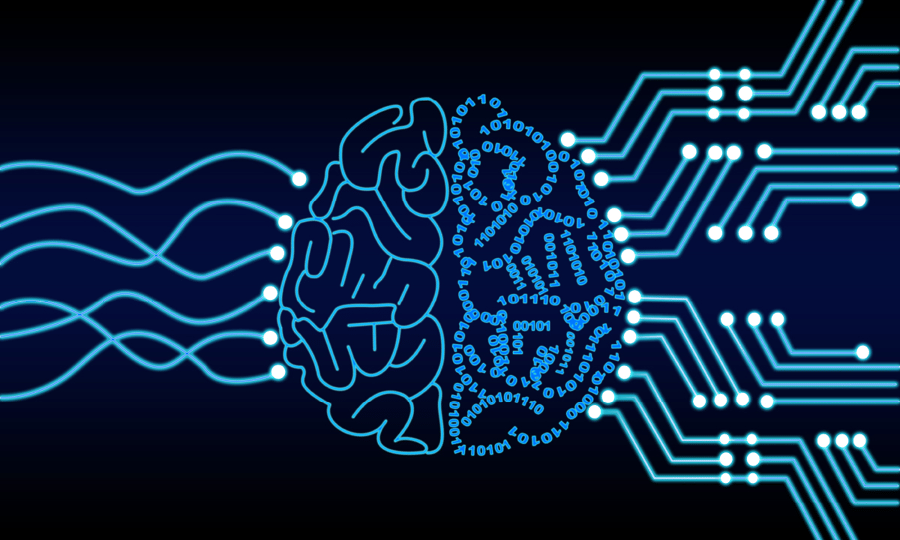
Many experts believe the next big trend in quality care is the use of clinical decision support (CDS) tools that are powered by artificial intelligence technologies. Hospitals, doctors, healthcare plans, medical researchers, drug companies, device manufacturers (among others) can use AI-fed CDS tools to prevent, detect, diagnose and treat acute and chronic diseases.
What “feeds” AI and its first cousin, machine learning? Simply put: structured clinical data and lots of it. Much like college students home on holiday breaks and ravaging through the refrigerator, both AI and machine learning have voracious appetites. A great source to feed those hungry technologies is your clinical quality measure data.
AI is any technology that mimics the human thought process. Machine learning, or ML, is one type of AI technology that learns and improves as it processes more data. For AI/ML technologies to consume and digest that data, it must be structured, according to the experts.
Why AI/ML Tech Prefers to Eat Structured Data
Structured data is data that “has been organized into a formatted repository, typically a database, so that its elements can be made addressable for more effective processing and analysis,” according to WhatIs.com, an information technology terminology website.
The challenge for developers of clinical AI/ML tech is that most clinical data is unstructured. It’s in different information systems. It’s in different formats. It’s in hand-written charts. It’s in lab reports. It’s in physician notes. It’s on different wearable devices. It’s in patient-reported outcomes. It’s in different computer languages. It’s in EHR systems that don’t “talk” to each other. It’s located across multiple clinical sites that cared for the same patient. It’s in the results of clinical trials sorted by different biopharma and device companies. And while sifting through unstructured data can uncover valuable insights, it’s challenging work that would add to both algorithm build costs and delivery timelines. Organizations need to evaluate whether this work will provide a good return on investment.
NAM: Everyone Must Bring Data to the Table
In December, the National Academy of Medicine (NAM), released a report about the potential and the pitfalls for clinical AI/ML technologies.
“The emergence of AI as a tool for better healthcare offers unprecedented opportunities to improve patient and clinical team outcomes, reduce costs, and impact population health,” the report said. But to realize those opportunities and achieve all those desired outcomes, “AI algorithms must be trained on population-representative data to achieve performance levels necessary for scalable ‘success.’”
The good news: “Trends such as the cost for storing and managing data, data collection via electronic health records, and exponential consumer health data generation have created a data-rich healthcare ecosystem.” The downside: “This growth in healthcare data struggles with the lack of efficient mechanisms for integrating and merging these data beyond their current silos.”
A Big Menu of Clinical AI/ML Tech Use Cases
In a report released last October, KLAS, the health IT market research and ratings firm, and CHIME (the College of Healthcare Information Management Executives), echoed NAM’s findings.
KLAS interviewed health IT executives at 57 healthcare organizations about their actual experiences in attempting to adopt AI/ML tech. The respondents identified 70 validated clinical use cases for AI/ML:
- 42 for population health
- 13 for clinical decision support
- 11 for clinical research
- Two each for patient engagement and clinical education
The KLAS/CHIME report provided examples of many of the 70 clinical use cases for AI/ML, including the prediction and prevention of:
- Emergency room visits
- Inpatient mortality
- Patient falls
- Pressure ulcers
- Readmissions
- Sepsis
“Don’t underestimate the time and effort it will take to prepare the data needed to test and build the (AI) models,” the report said. “Healthcare data is hard to clean and comes from many sources, and your organization may not have the expertise to feed the right variables or features into your models.”
A Rich Source of Data for Your AI/ML Tech
You don’t have to be a data scientist to see the parallels between many of the clinical use cases and the clinical quality measure data that your hospital, health system or medical practice collect, aggregate, and then report to Medicare and other payers as part of their myriad value-based reimbursement programs.
It seems that clinical AI/ML tech developers, whether they’re in-house or third-party vendors, need what you have: lots of structured clinical data. And it seems that you need what clinical AI/ML tech developers have: lots of CDS tools to prevent, detect, diagnose and treat acute and chronic diseases.
Chances are, your healthcare organization is thinking about adopting or starting to adopt AI/ML tech to improve the quality of patient care that you provide.
Twenty-two percent of senior-level healthcare executives at 128 organizations recently surveyed by HealthLeaders said they have AI/ML software capabilities now, and another 31% said they intend to have such capabilities within the next three years. Eighty-one percent said the data that they feed or intend to feed into their AI/ML software is clinical data. Financial data came in second at 72%.
Eighty-six percent of the HealthLeaders survey respondents work in a health system, hospital or medical practice. If you’re a part of the respondents that have AI/ML software capabilities, take the opportunity to reach out to your quality department. Connect with the chief AI officer, data scientists, AI engineers, data engineers, data governance experts and data entry experts at your organization to learn how your clinical quality measure data —your bread and butter—can feed their AI/ML algorithms.
|
Comments